Black-Box Explanation of Object Detectors via Saliency Maps (D-RISE)
Vitali Petsiuk, Rajiv Jain, Varun Manjunatha, Vlad Morariu, Ashutosh Mehra, Vicente Ordonez, Kate Saenko
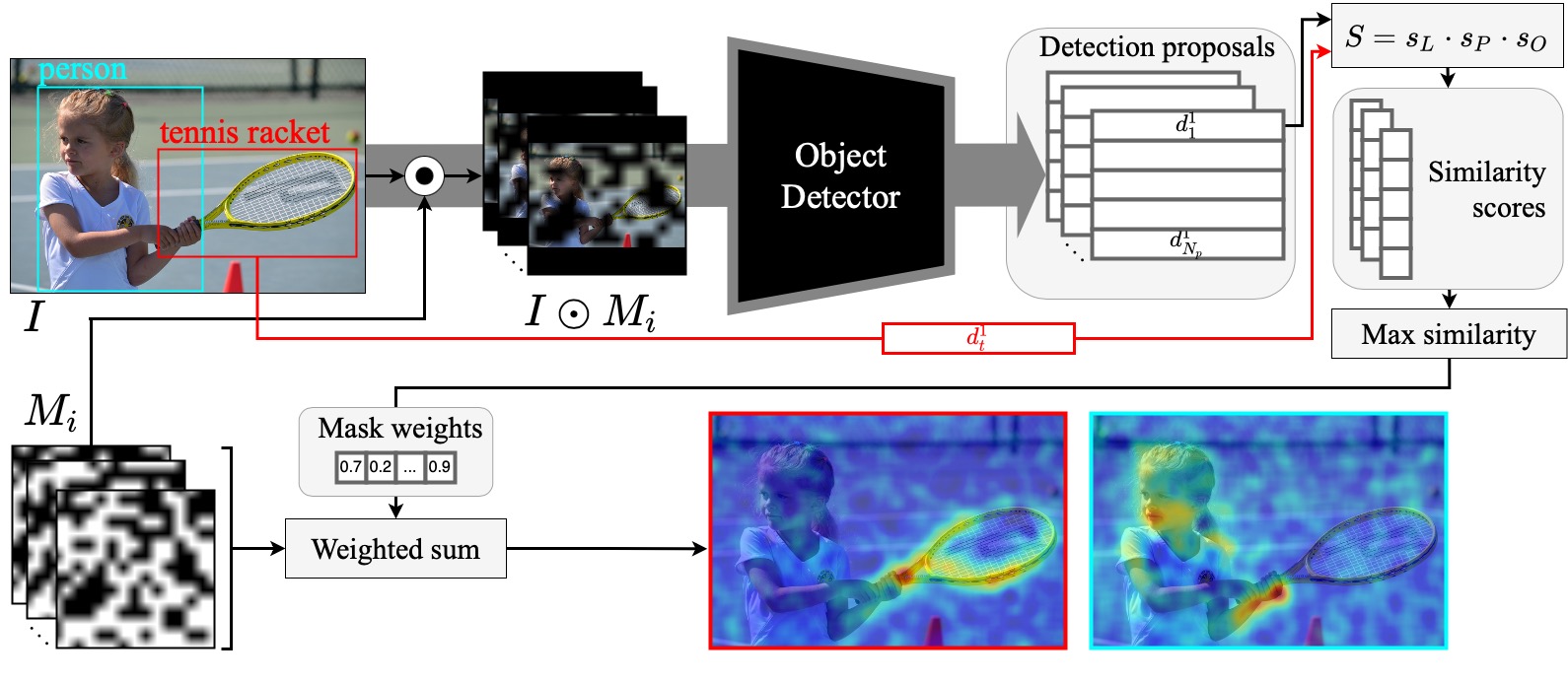
Abstract
We propose D-RISE, a method for generating visual explanations for the predictions of object detectors. Utilizing the proposed similarity metric that accounts for both localization and categorization aspects of object detection allows our method to produce saliency maps that show image areas that most affect the prediction. D-RISE can be considered “black-box” in the software testing sense, as it only needs access to the inputs and outputs of an object detector. Compared to gradient-based methods, D-RISE is more general and agnostic to the particular type of object detector being tested and does not need knowledge of the inner workings of the model. We show that D-RISE can be easily applied to different object detectors including one-stage detectors such as YOLOv3 and two-stage detectors such as Faster-RCNN. We present a detailed analysis of the generated visual explanations to highlight the utilization of context and possible biases learned by object detectors.
Slides
Presentation (CVPR, 2021)
Bibtex
@inproceedings{Petsiuk2021drise, author = {Vitali Petsiuk and Rajiv Jain and Varun Manjunatha and Vlad I. Morariu and Ashutosh Mehra and Vicente Ordonez and Kate Saenko}, title = {Black-Box Explanation of Object Detectors via Saliency Maps}, booktitle = {Computer Vision and Pattern Recognition (CVPR)}, year = {2021} }