Exploiting phonological constraints for handshape recognition in sign language video
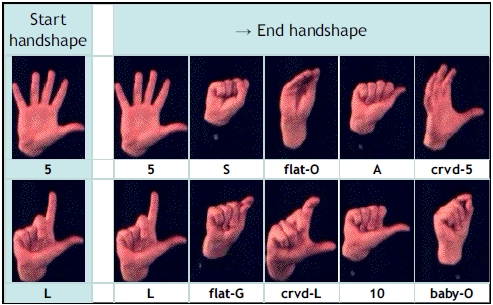
|
Handshape is a key articulatory parameter in sign language and thus
handshape recognition from signing video is essential for sign recognition and retrieval.
Handshape transitions in lexical signs (the largest class of signs in signed languages)
are governed by phonological rules that constrain the transitions to involve either
closing or opening of the hand (i.e., to exclusively use either folding or unfolding
of the palm and one or more fingers). Furthermore, akin to allophonic variations in
spoken languages, variations in handshape articulation are observed among different
signers. We propose a Bayesian network formulation to exploit handshape co-occurrence
constraints and utilizes information regarding allophonic variations to aid handshape
recognition. We propose a fast non-rigid image alignment method to gain improved
robustness to handshape appearance variations during computing observation likelihoods in
the Bayesian network. We evaluate our handshape recognition approach on a large corpus of
lexical signs (described in a subsequent project below). We
demonstrate improved handshape recognition accuracy leveraging linguistic constraints
on handshapes.
[CVPR2011 paper (to appear)]
|
Learning a family of detectors via multiplicative kernels
|
Object detection is challenging when the object class exhibits large
within-class variations. In this work, we show that foreground-background classification
(detection) and within-class classification of the foreground class (pose estimation)
can be jointly learned in a multiplicative form of two kernel functions. Model training
is accomplished via standard SVM learning. Our approach compares favorably to existing
methods on hand and vehicle detection tasks.
[T-PAMI 2011 paper]
[CVPR 2008 paper]
[CVPR 2007 paper]
|
Layers of graphical models for tracking partially-occluded objects
|
We propose a representation for scenes containing relocatable objects
that can cause partial occlusions of people in a camera's field of view. In this
representation, called a graphical model layer, a person's motion in the ground plane
is defined as a first-order Markov process on activity zones, while image evidence
is aggregated in 2D observation regions that are depth-ordered with respect to the
occlusion mask of the relocatable object. The effectiveness of our scene representation
is demonstrated on challenging parking-lot surveillance scenarios.
[CVPR2008 paper]
|
Large video corpus for American sign language retrieval and indexing algorithms
|
There is currently a dearth of large video datasets for sign language
research, most notably, those that include variations among different native
signers, as well as linguistic annotations pertaining to phonological properties
for different articulatory parameters such as handshape, hand location, orientation,
movement trajectory and facial actions. Towards bridging this gap, we introduce the ASL Lexicon Video Dataset, a large
and expanding public dataset containing video sequences of approximately three thousand
distinct ASL signs produced by three native signers. The dataset includes annotations
for start/end video frames, gloss label for every sign (an English desctiptor label
for the sign) and start/end handshape labels. These annotations were coded using
SignStream. A
portion of the dataset corresponding to lexical signs used in
our handshape recognition project described above is displayed here.
[CVPR4HB2008 paper]
|
|